Decaf vs. regular coffee blinded experiment
Table of Contents
Abstract #
I conducted a two-week blinded, randomized experiment to test whether drinking regular vs. decaf coffee had a detectable effect on my mood and alertness. Each day, I drank one cup of coffee in the morning and immediately took a Quantified Mind test. I also tracked hours of sleep each night and measured subjective factors such as mood and alertness a few times throughout the day. Overall, the experiment was a success in terms of being able to follow my original plan. It did end up being only mostly blinded because I got caffeine withdrawal headaches after two consecutive decaf days.
Key Results #
Note: If you want to have the story told in an order that’s faithful to the chronology, skip this section and go straight to Introduction.
- Caffeine consumption meaningfully affected my mood but didn’t significantly improve any of my Quantified Mind sub-test scores or my subjective productivity. While this may be because this experiment is statistically under-powered, from a decision-theory perspective, it’s satisfactorily answered my original question.
- Although I didn’t randomize sleep (so results to be taken with a grain of salt), I found that sleep duration also, surprisingly, had non-significant impact on all of my Quantified Mind scores with the exception of Visual Backwards Digit Span. This suggests doing a follow-up experiment that attempts to directly measure the effect of sleep on cognitive performance would be worthwhile (similar to this one).
- Introspection is as miscalibrated as everyone talks about. I’d repeatedly go to do my Quantified Mind on days on which I felt crappy and be surprised to see my results were basically the same as days on which I’d felt good.
More discussion of these results can be found in the Qualitative and Quantitative results sections.
Introduction #
For a while, I’ve been convinced that decaf coffee has roughly the same effect on me as regular coffee. However, I haven’t been able to say with certainty because there’s huge potential for placebo effects. Starting tomorrow, I’ll be conducting a (99%) blinded experiment to test whether drinking regular vs. decaf coffee has a detectable effect on my mood and alertness.
I intend to record the metrics I describe in the next section under the ‘Data collection’ heading of this post and will also report results here once the experiment is over. I’ll make data from my Quantified Mind experiment (discussed below) available as well in CSV format.
See something wrong with this experiment plan, my analysis, and/or my results? Email me at first.last+blog-at-gmail.com (see top left header for spelling) or comment on this post!
Experiment #
Preparation #
To prepare for the experiment, I split 2 weeks’ worth of coffee (7 days of decaf, 7 days of regular) into 14 bags. After I split the coffee into 14 bags, my uniquely wonderful lab assistant (girlfriend) sorted them into a random order (with labels for each day) by flipping a coin to decide whether that day’s coffee would be regular or decaf.
Procedure #
Starting tomorrow, for the next 14 days, I’ll make my coffee using the grounds from the labeled bags and track a few subjective metrics three times a day (right after having coffee, at 1 PM, and at 6 PM):
- Alertness (1-5 scale): loosely defined as how tired and sleepy I feel.
- Sharpness (1-5 scale): the opposite of ‘fogginess’.
- Mood (1-5 scale): a coarse-grained measure of how ‘good’ I feel emotionally.
- Headache (yes/no): I get headaches if I don’t have coffee in the morning, so it will be interesting to see whether I get them on decaf days even when I don’t know the coffee is decaf.
- Regular vs. decaf day (regular/decaf): “Given how I feel today, do I think this morning’s coffee was regular or decaf?”
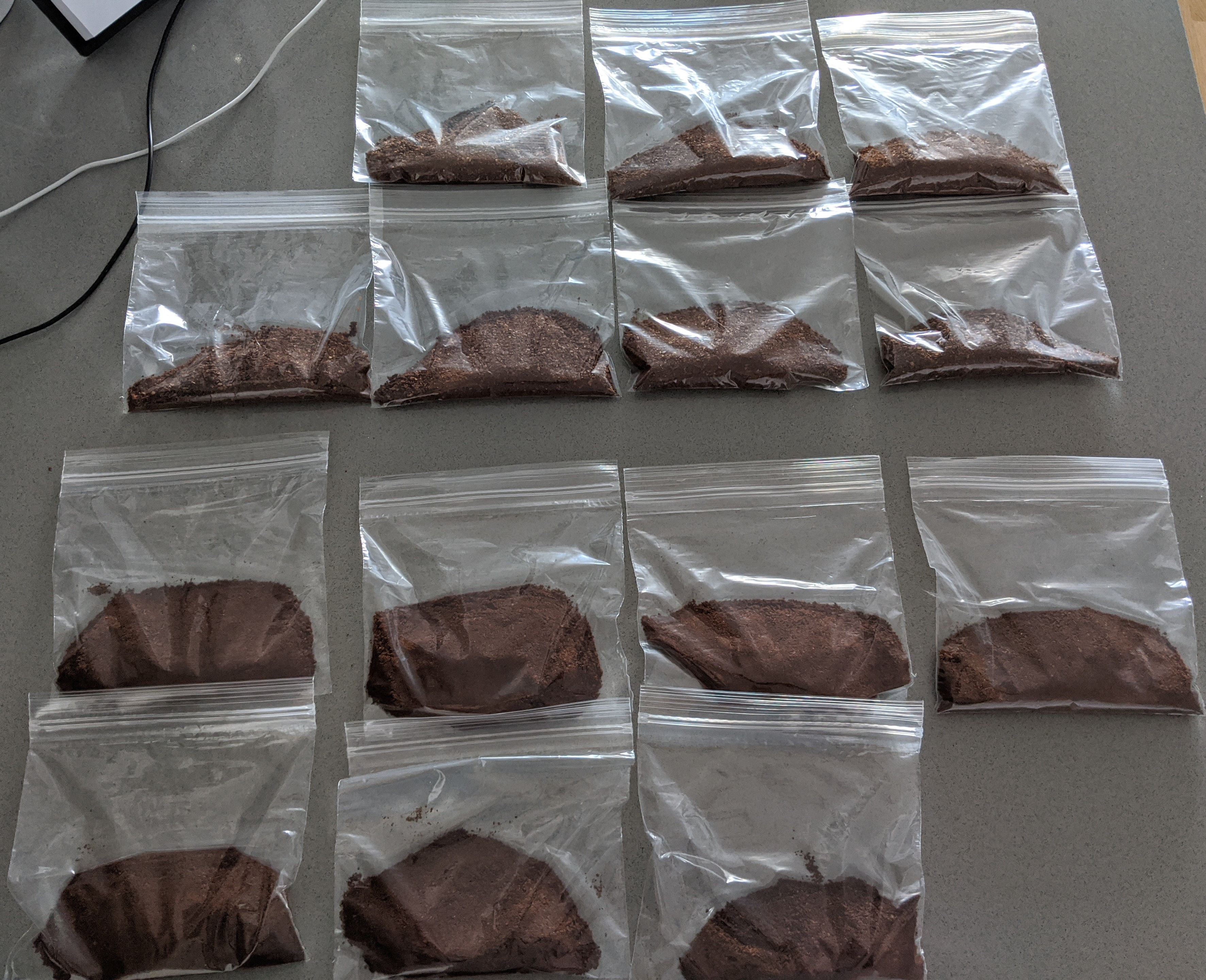
I’ve also set up a Quantified Mind (QM) experiment which will give me 8 minutes of cognitive tests each day and record my scores. I’ll take these tests immediately upon finishing my coffee in the morning.
Materials #
I’m using Swiss Water’s version of Joe Coffee Nightcap Decaf and Joe Coffee Colombia La Familia Guarnizo regular coffee both brewed in a Mr. Coffee drip coffee maker. I chose Swiss Water for decaf after reading that they do the best job of filtering out >99% of the caffeine from the beans.
For cognitive tests, I’m using QM’s 8 minute “coffee” test that includes tests of executive function, working memory, and visuospatial something.
Confounders #
Sleep #
Getting less than 7 hours of sleep affects how sharp I feel throughout the day and how awake I feel in the afternoon. Even though this is a randomized experiment, 14 days is short enough that were my sleep schedule to get interrupted, noise from sleep quality variance could easily overwhelm the signal from drinking regular vs. decaf coffee in the data. I plan to deal with this in two ways:
- Track how much sleep I get each night (in number of hours). Unfortunately, I don’t have a good sleep tracker so this will just be based on estimate of when I went to bed, how long it took me to fall asleep, and when I woke up.
- Avoid major sleep disruptions and sleep roughly the same number of hours each night. That said, if 2 fails and my sleep schedule gets messed up during the experiment, I’ll be much less confident in the results.
(ETA on 03/02.) In the comments, Bucky points out that caffeine use may also impact sleep the next night, making the confounding even more complex. My current plan is to test for evidence of this when I do my analysis. Pre-registering that I’ll be surprised if my one cup of coffee in the morning has much of an impact, but being surprised is the whole point of something like this!
Diet #
Relative to sleep, I’m less convinced that regular dietary variation–i.e. eating relatively ‘healthy’ food and not being moribdly obese–has much of an effect on cognitive performance. But I still will keep my regular eating schedule of skipping breakfast and only having lunch and dinner as I suspect this will also help me keep a regular sleep schedule. To keep myself honest here, I’ll track when I eat each day.
Internet Use #
(ETA on 03/02.)
I know this one seems weird but anecdotally, I’ve found procrastinating on the more addicting internet website (read: Twitter) causes me to feel a lot fuzzier for the rest of the day.
Caffeine Withdrawal #
(ETA on 03/02.)
In the comments, Issa Rice points out that caffeine withdrawal may begin anywhere between 12 and 24 hours after not having caffeine and peaks around 50 hours. This means that depending on the order of consecutive days, I may or may not go through full withdrawal, which would presumably impact my results.
Mood #
(ETA on 03/02.)
In the comments, Pattern points out that mood and events that affect it might affect the results. I’ve added a mood metric to my list of subjective metrics to track to prepare for this possibility.
Cognitive test practice effects #
Given that I haven’t been doing QM tests before the experiment to calibrate, there’s a risk of practice effects dominating differences between caffeine and no caffeine days. I’m not totally sure how to deal with this yet, but isn’t this the use-case for random effects regressions?
Analysis #
On not pre-registering in detail #
Since I’ve been reading Gelman’s wonderful Bayesian Data Analysis and also view this study as a good candidate for a Bayesian approach due to the experiment having a small \( n \), I intend to use Bayesian methods for my analysis. In an ideal world, I’d pre-register exactly what analyses I intend to do now (as of 03/01), but unfortunately, I’m still enough of a noob at this that I need to spend a good chunk of time reading about the right way to set up the analysis. For now, I’m recording the questions I want to answer below and will edit to add details of the analysis as I figure them out.
I worry less than I normally would about post-hoc changing the analysis to find a significant result because I don’t have strong incentives to find one. That is, I’m genuinely interested in the ’true’ answer to the question and don’t have a strong desire for it to be ’there’s a big effect’ or ’there’s no effect’. Being transparent about the results of each stage of analysis should also help keep me honest. (Of course, I could always post-hoc choose not to share intermediate stages but again I don’t think my incentives are to do that.)
High level plan #
At a high level, I want to test the effect of regular vs. decaf coffee on alertness, sharpness, headaches, and my QM results. This is complicated by the fact that my prior is that the response variables I described above only share some common causes and that the causal effects of caffeine consumption differ between the response variables. For example, I suspect alertness and QM test scores are both affected by sleep quantity and coffee consumption but that alertness may also be impacted by other confounding variables like mood and plans for the day.
To mitigate this, I’ll heavily rely on the most objective response variable, the QM results, to determine the magnitude of the ’true effect’. In causal terms, this is equivalent to assuming that sleep is sufficient for blocking all ‘backdoor’ paths between regular vs. decaf coffee and cognitive ability. I’m still measuring the other subjective variables because I’m curious to see how correlated they are with my QM results and each other and other want to leave open the possibility of doing other analyses that come to mind and seem interesting.
FAQ #
This is currently (as of 03/01) a list of questions that I came up with for myself, but I’ll also add answers to questions others raise in this section.
Isn’t this too short? #
As I mentioned, 14 days is short enough that even though the regular vs. decaf day assignments are randomized and blinded, the ‘statistical power’ of my results will be relatively weak. Two responses to this:
- From a decision-theoretic perspective, I mostly care about the easier to answer question of was the effect meaningful enough that I could accurately detect whether the coffee I had that day was regular or decaf conditional on what I know about my sleep and other factors.
- I’m going to use Bayesian methods and will be more than willing to label the results ‘inconclusive’ if my analysis results in a diffuse posterior.
Why ‘99%’ blinded? #
I’m calling this 99% blinded because there is a slight visual difference between the two coffee grounds that I could in theory detect while making my morning coffee. By making my coffee in the dark (I do this already) and having the bags pre-sorted so I barely have to look at them, I hope to minimize the likelihood of ‘de-blinding’ the experiment. I tried to minimize the likelihood further by buying identical decaf and regular grounds but unfortunately couldn’t find a seller that sold the same beans in decaf and regular. In lieu of that, I settled for buying beans from the same region with the same flavor profile (I also don’t have very good taste sense) so as to limit the difference to a visual one.
Data Collection #
Recording subjective metrics and sleep duration in this Google spreadsheet (to make export to CSV easy.
Results #
Qualitative #
Note: I originally wrote this section soon after I finished the experiment but for whatever reason never posted it here.
I’m done! Made it through the withdrawal headaches. I haven’t done much analysis yet but here are a few of my initial observations, some of which I won’t be able to verify with analysis.
- I did pretty well at identifying which days were caffeine vs. decaf days. I only made two mistakes and one of them I had a hunch I was wrong in hindsight.
- Decaf days affected my actual subjective productivity less than expected. The main beneficial effect of caffeine seemed to be that it lowered the activation energy for me to get started on tasks and on days in which I’d slept well seems to add a certain ‘sharp’ quality to my thinking.
- Sleep matters for subjective measurements but not necessarily for the performance metrics I looked at. See the analysis section for further discussion of the latter point. On the former point (subjective measurements), anecdotally, especially if we ignore the headaches (which were a result of withdrawal not drinking decaf coffee in general), the difference in all my subjective metrics seemed to correlate much more with how much sleep I got before than with regular vs. decaf coffee.
- Caffeine may not help me do better when sleep deprived. As mentioned above, I do notice a small subjective positive effect on my ‘sharpness’ when I sleep really well, have caffeine, and fast (which I do most days until lunch). On the other hand, on days on which I got <7 hours of sleep (happened before both caffeine and decaf days), I felt like caffeine either made no difference or made me a bit more awake at the cost of making my cognition even fuzzier. I highly doubt this will show up in the Quantified Mind metrics in any detectable way but I wanted to note it as a hypothesis that I’m very interested in as part of my general interest in mitigating the effects of sleep deprivation.
- (Credit to Issa Rice for pointing out that this would be an issue when I proposed the experiment.) Withdrawal did turn out to be a bit of an issue although not enough of one (IMO) to mess up the results of the experiment. My first decaf sequence was two days in a row and in the afternoon I got a bad withdrawal headache that was resistant to Ibuprofen. On later decaf days, I took Ibuprofen at the first sign of a headache and this seemed to largely mitigate withdrawal symptoms. Of course this does confound my headache tracking a bit, but I view it as worth it in order to try minimize the effect of withdrawal on other metrics.
Quantitative #
Unfortunately, due to time constraints and also an informal value-of-information calculation, I did limited analysis of my results data, mainly just looking at two basic relationships: the effect of regular vs. decaf coffee on my Quantified Mind metrics and the relationship between sleep duration and my Quantified Mind metrics. If you’re interested in my full analysis, you can find the HTML output from my Jupyter notebook here. While I’d still like to do some Bayesian analysis of the data, I’m considering this as “done” without it.
Before I discuss how I analyzed my results, I want to explicitly note some assumptions I made and things I failed to look at:
- Assumed approximately linear relationships between sleep duration and the scores I looked at.
- Didn’t model interaction effects, meaning that for example, I have no way to know whether sleep somehow interacting with caffeine affected the results.
- Used null hypothesis significance testing despite generally being a proponent of Bayesian methods.
As I’ve emphasized, given the small number of data points I collected, doing the additional analyses to investigate or remove these assumptions didn’t seem worth it. But, if I were to do another follow-up experiment, I would try and explicitly model the generative process of my data, use posterior probabilities, and maybe look at non-linear effects between covariates.
Now, we can actually talk about results!
Caffeine vs. Decaf Coffee had essentially no effect on my Quantified Mind sub-test scores #
First I looked at how my Quantified Mind scores varied between caffeine and decaf days. The below graph shows violin plots comparing each test’s scores for days on which I had regular vs. decaf coffee respectively. As you can pretty easily see, the means of the respective groups are, in almost every cases very (not significantly as measured by a paired two-sample t-test) close. The only case in which they differ meaningfully (and barely significantly with p = .049, which we all know is a sign that the hypothesis you’re testing is definitely true for all of eternity) is “Visual Backward Digit Span”. Unexpectedly, in this case, I seem to do better when not caffeinated than when caffeinated. That said, as I hinted at with my parenthetical, I’ve read enough Andrew Gelman blog posts to not put much stock in the weak “significance” result on its own. On seeing this initially, I realized that even if the correlation is meaningful, my experiment was short enough that sleep could be the real causal factor here. The main takeaway for me from this set of plots / analysis is that regular vs. decaf coffee doesn’t make a big enough difference in my cognitive performance to show a meaningful effect within two weeks.
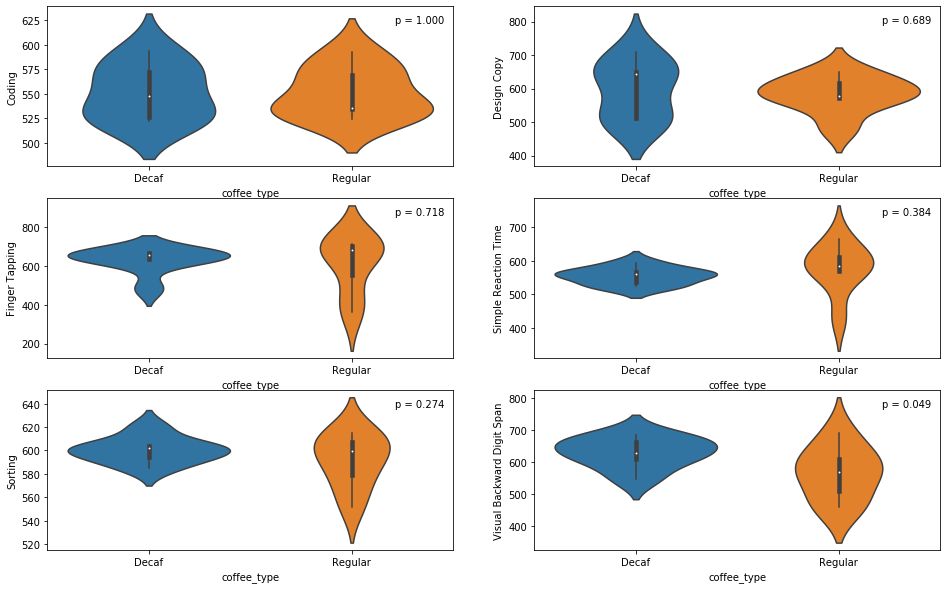
Sleep duration only meaningfully correlated with 1 one out of 6 Quantified Mind sub-tests #
Curiosity about whether sleep duration influenced my scores led me to the second visualization / statistical analysis I did. Before I go on, I want to add the caveat that I wasn’t randomizing my sleep so any results from this section inherit all the standard issues of inferring things from observational data. Personally, I think of these results as at best hinting at worthwhile follow-ups rather than as telling us much about the effect of sleep cognitive performance. Caveats aside, the following graph shows the best-fit (OLS) regression lines for each Quantified Mind test score as a function of sleep duration. As you can see, outside of “Visual Backward Digit Span”, all the lines have slopes that are quite close to 0 (and have 95% confidence intervals that include 0). This suggests that it might be worth me running a longer experiment where I randomize (but obviously can’t blind) sleep duration and then do, in particular working memory heavy, Quantified Mind tasks.
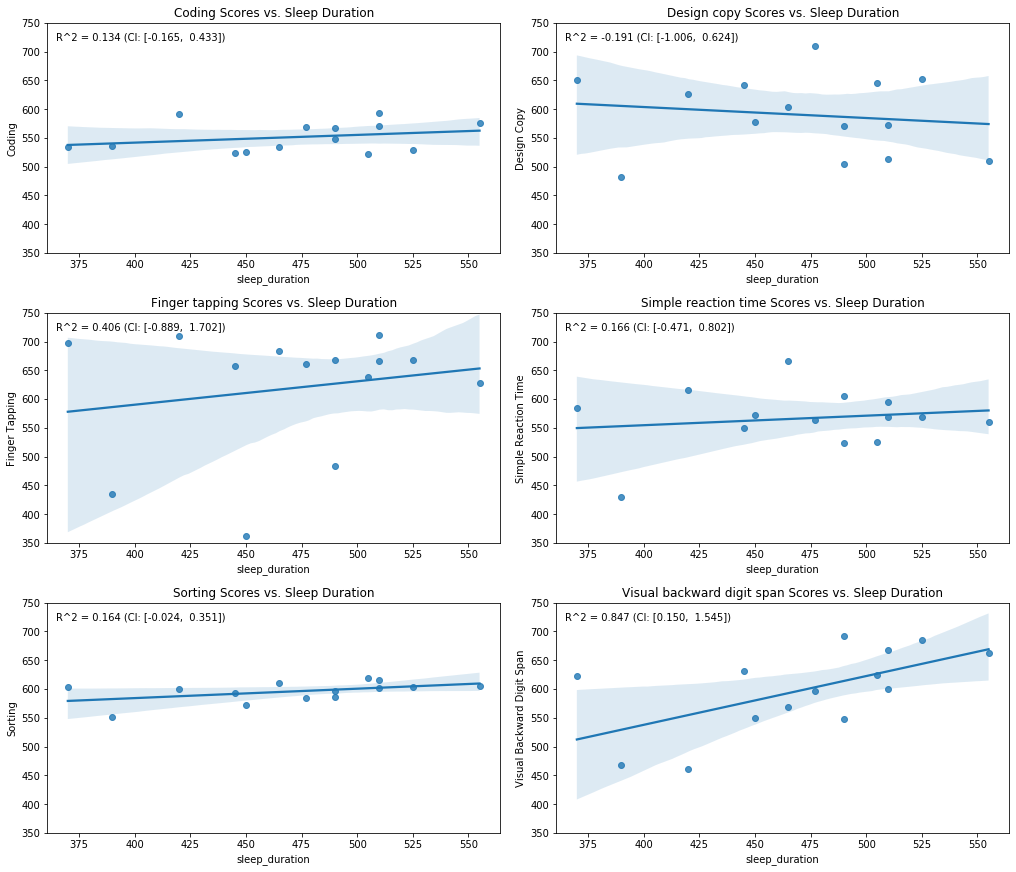
Practice effects likely affected scores at least 2 of the 6 Quantified Mind sub-tests #
Last, I briefly looked at my scores as a function of date to see whether practice effects were an issue. From my very basic linear regression analysis below, it appears that practice effects may have been a factor, in particular for finger tapping (which matches my subjective perception of which things I got better at). What’s interesting is that it seems least likely that practice effects were an issue for the digit span task, but that’s the only task where I found differences in terms of other covariates.
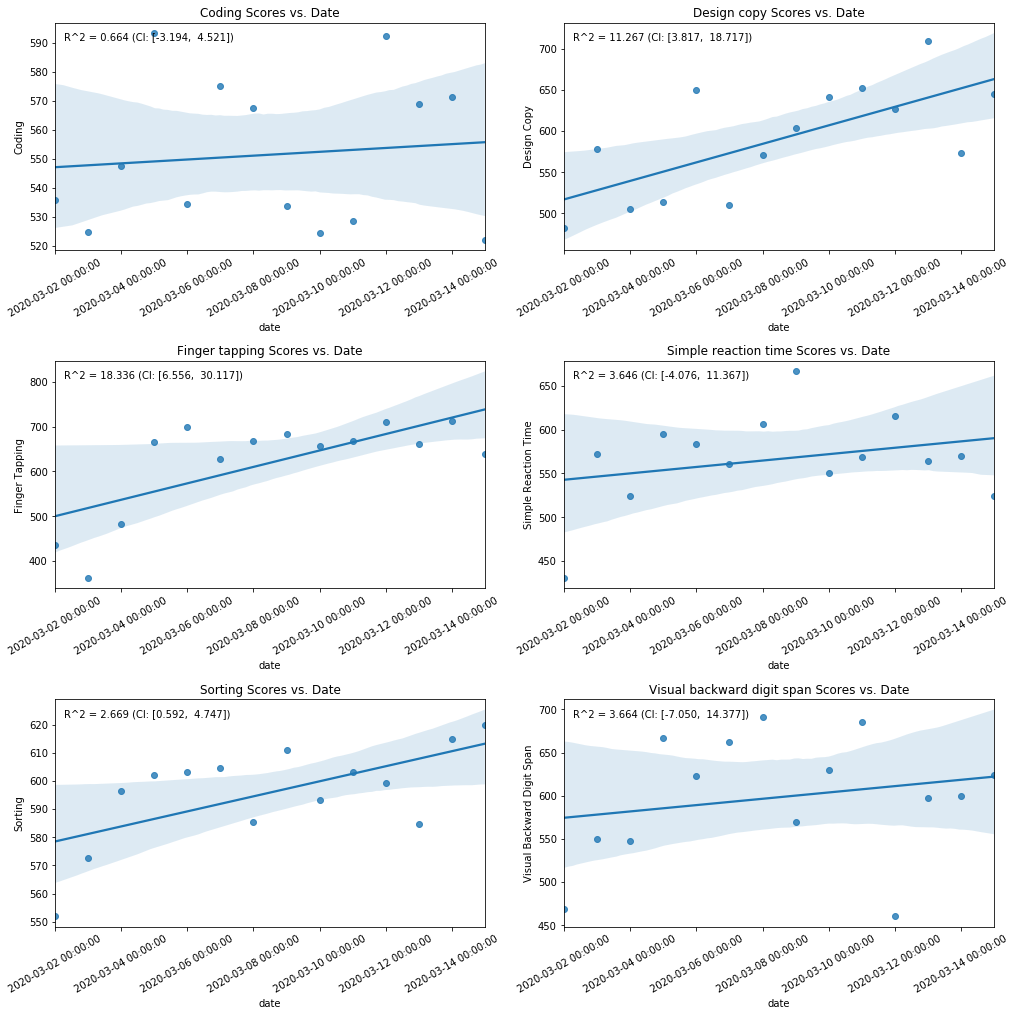
To summarize, my conclusion from this analysis is that having decaf vs. regular coffee doesn’t seem to have enough of an impact on cognitive performance to show up in a 2-week randomized Quantified Mind experiment. On one hand, it doesn’t seems like sleep has a massive effect either. But, taking into account that the sleep data was observational and that sleep duration did correlate with performance on backwards digit span, a longer experiment on sleep’s effect on performance metrics might be warranted. Were I to do one, I’d pair it with a more rigorous analysis.
Takeaways #
My object-level takeaways from running this experiment and analyzing the data are:
Object Level #
- Detoxing from coffee sucks from a happiness perspective but doesn’t seem too bad from a mental performance perspective.
- Introspection is as miscalibrated as everyone talks about. I’d repeatedly go to do my Quantified Mind on days on which I felt crappy and be surprised to see my results were basically the same as days on which I’d felt good.
Meta Level #
- With respect to the analysis, I was reminded of Hofstadter’s Law for the hundredth time.
It always takes longer than you expect, even when you take into account Hofstadter’s Law.
- Being in a relationship is a great way to always have someone to help you conduct blinded, randomized experiments (and also is great for me in general!).